According to our Consumer Pulse observatory, 63% of interviewees report a reduction in their savings capacity and 34% register increased debt levels for two consecutive years. This last portion reports a significant reduction of perceived well-being of 21%.
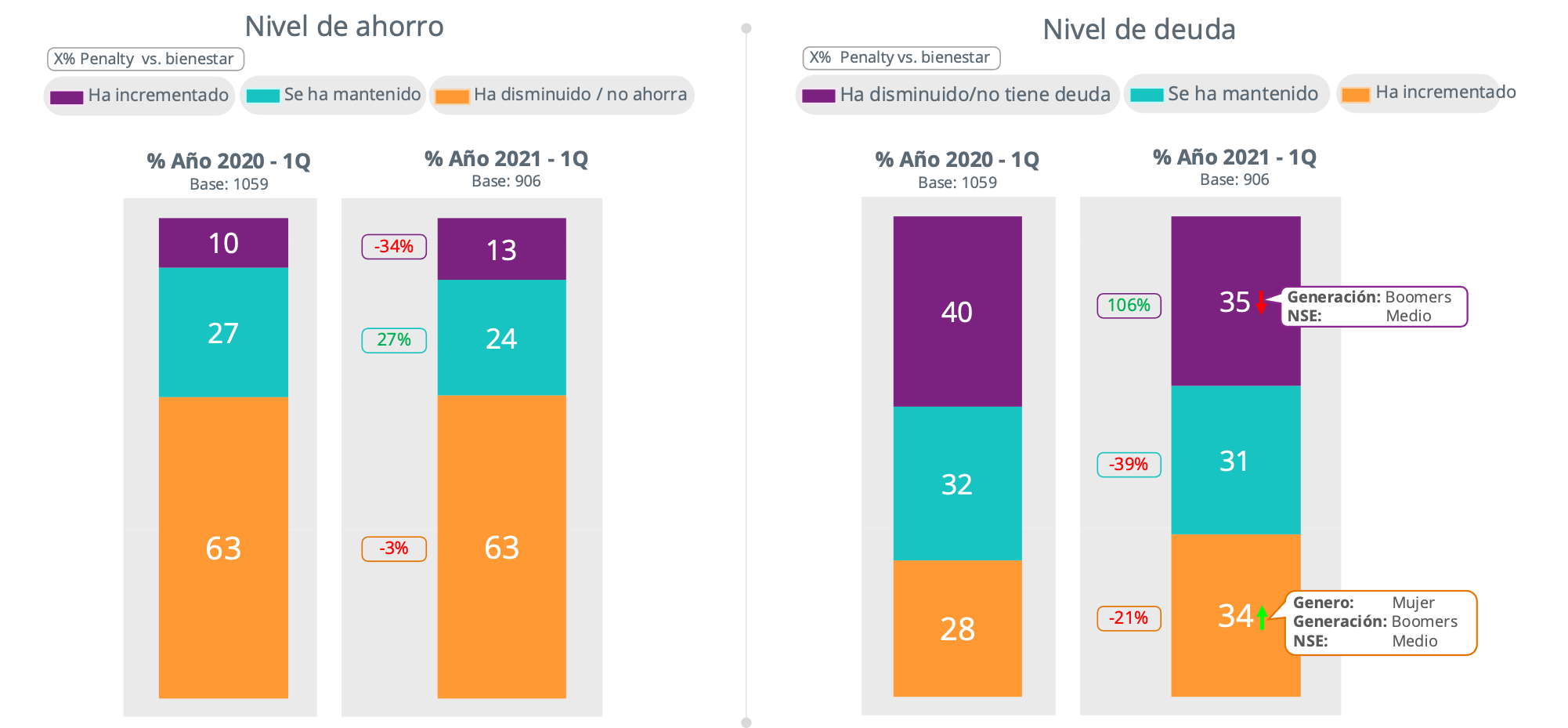
This indicates that the economic tension resulting from the pandemic could be posing challenges of debt payment capacity in consumers; the payment delinquency phenomenon extends to commercial loans.
Collection is a key contact point that is rarely shown in the Customer Journey Map. Depending on how this is done, it could generate problems for the customer experience. The purpose of this blog is to analyze best practices with respect to “Predictive Collection” and suggest some ideas that stem from our consulting experience with BPO companies (Business Process Outsourcing), banks and insurance companies.
BEST ANALYTICS AND DIGITAL PRACTICES IN COLLECTION PROCESSES
Analytics applied to collection processes could be represented by a metaphor of a triangle with three vertices.
Collection function Generation of process data and results with analytical purpose.
Data-centric Technology favoring push and pull strategies during the process.
Operational excellence Integrating operations and optimization research to the collection process.
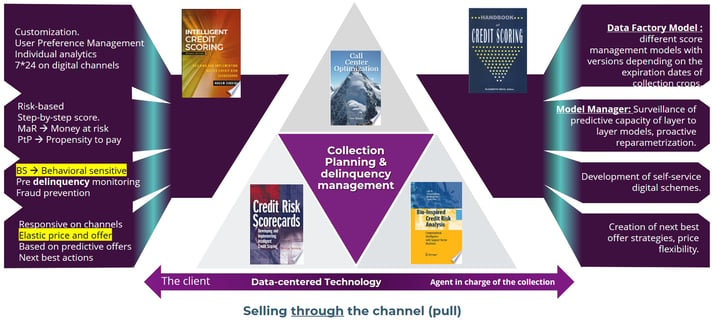
The Collection function
Characteristic features that indicate trends from the following perspective:
Creation of 7 * 24 self-managed channels to capture customer data. From this perspective, the customer can auction payment options according to his particular possibilities.
Auction and price optimization algorithms would be scheduled for these self-managed platforms. These algorithms take advantage of price sensitivity analytics to estimate the optimal level of debt reduction needed to motivate the payment without “leaving money on the table”.
MaR (Money at Risk) and PtP (Propensity to Pay) models to support collection decisions and trigger user campaigns.
Behavioral sensitive: Automatized collection actions, as well as the models, must be aimed at the behavioral changes of the user. Transactional systems would have to capture these behaviors using monitoring technologies and establishing the user’s Empirical Journey.
Next Best Action: In accordance with the price elasticity (Minimum reduction of the obligation needed to raise the PtP ("Propensity to pay") models must be programmed in order to propose actions to the user, motivating their payment behavior. In this sense, advances in behavioral economics with particular emphasis on "Nudge" would support the process motivationally.
Operational excellence.
A common denominator in collection processes is that they excel at storing results (collection), but are not very efficient in storing process data; generally, the process lacks variables such as:
Time between processes
Notes from the collection analyst
Information from the “People analytics” agent
Omnichannel information - chat, bots and WhatsApp-
Generally, the process is optimized rather than the result; without data thereof, it is impossible to achieve optimization. In this sense, an essential part of creating a “data-centric” pro “Customer Experience” collection model is to collect process information consistently.
As a result of our consulting experience with BPO companies, banks, insurance companies and other companies in the financial sector, we have built a roadmap of the collection process’ analytic evolution, avoiding affecting the user experience as shown below:
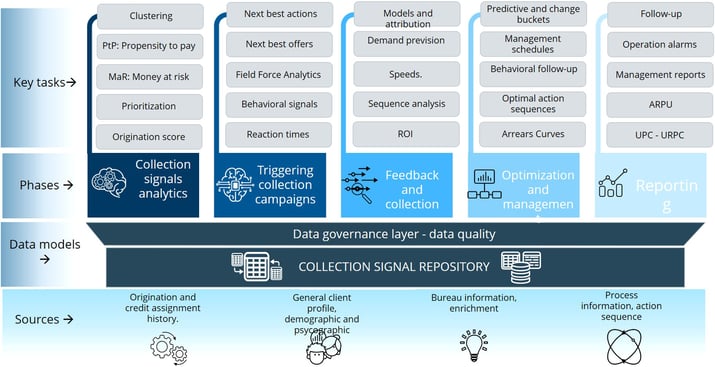
The operation and self-managed data are captured in a data model we call COLLECTION SIGNAL REPOSITORY. A governance and data quality layer is deployed over this data model to feed five core task nuclei:
Collection signals analytics
Triggering collection campaigns
Feedback and collection
Optimization and management
Reporting
We believe we can add value to your collection strategy by integrating market research, data analytics and artificial intelligence. We will contact you according to your availability
South America
Mexico and Central America